This is my reading notes for Chapter 6 in book “System Design Interview – An insider’s guide (Vol. 1)”.
Table of Contents
Introduction
Chapter 6 delves into designing a key-value store, a fundamental building block in distributed systems. Key-value stores are pivotal for applications requiring low-latency data access, high availability, and scalability. The chapter explores various aspects of key-value store design, from basic concepts to advanced techniques used in large-scale distributed systems.
Key Concepts and Basic Operations
- Key-Value Pair:
- A key-value store uses a simple yet powerful structure where data is stored as pairs of keys and values. The key is unique and can be used to retrieve the associated value efficiently.
- Example:
- Key:
"user_12345"
- Value:
{"name": "John Doe", "email": "john.doe@example.com", "last_login": "2024-08-11T10:00:00Z"}
- Key:
- Supported Operations:
- Put (key, value): This operation inserts or updates the value associated with the key.
- Example:
put("user_12345", {"name": "John Doe", "email": "john.doe@example.com"})
inserts the user information.
- Example:
- Get (key): This operation retrieves the value associated with the key.
- Example:
get("user_12345")
returns{"name": "John Doe", "email": "john.doe@example.com"}
.
- Example:
- Put (key, value): This operation inserts or updates the value associated with the key.
Design Scope and Considerations
- Design Requirements:
- The key-value store should be capable of handling millions of read and write requests per second while ensuring high availability, low latency, and scalability.
- Scalability: The system should be able to scale horizontally by adding more nodes.
- Consistency: The design should balance between consistency, availability, and partition tolerance (CAP theorem).
- Trade-offs in Design:
- Consistency vs. Availability: In the face of network partitions, a trade-off must be made between consistency (all nodes see the same data) and availability (the system is always available).
- Example: Dynamo prioritizes availability and partition tolerance over strict consistency, leading to eventual consistency.
Single Server Key-Value Store
- In-Memory Hash Table:
- A simple implementation uses a hash table where keys are hashed to determine the location of their corresponding values in memory. This allows for constant time complexity (
O(1)
) for both put and get operations. - Limitations: The in-memory approach is constrained by the amount of available memory and lacks durability (data is lost on server failure).
- A simple implementation uses a hash table where keys are hashed to determine the location of their corresponding values in memory. This allows for constant time complexity (
- Optimizations:
- Data Compression: Compressing values can save memory space, but this introduces additional computational overhead during compression and decompression.
- Memory Management: Implementing a Least Recently Used (LRU) cache can keep frequently accessed data in memory, while less frequently accessed data is persisted to disk.
Distributed Key-Value Store
- Motivation for Distribution:
- To handle large datasets and provide high availability, the key-value store must be distributed across multiple servers. This distribution introduces challenges such as data partitioning, replication, and ensuring consistency across nodes.
- CAP Theorem:
- The CAP theorem states that a distributed system can only provide two out of three guarantees: Consistency, Availability, and Partition Tolerance. This leads to different design strategies:
- CP Systems: Prioritize Consistency and Partition Tolerance (e.g., HBase).
- AP Systems: Prioritize Availability and Partition Tolerance, accepting eventual consistency (e.g., Cassandra, Dynamo).
- The CAP theorem states that a distributed system can only provide two out of three guarantees: Consistency, Availability, and Partition Tolerance. This leads to different design strategies:
System Components and Techniques
- Data Partitioning:
- Consistent Hashing: Used to evenly distribute data across nodes. Consistent hashing minimizes data movement when nodes are added or removed, making the system more scalable and fault-tolerant.
- Example: In a consistent hashing ring, servers (nodes) and keys are both hashed to a position on the ring. A key is assigned to the first server encountered as you move clockwise around the ring.
- Data Replication:
- To ensure availability and durability, data is replicated across multiple nodes.
- Replication Factor (N): The number of copies of each piece of data.
- Quorum Mechanism: Used to achieve a balance between consistency and availability.
- R (Read Quorum): The minimum number of nodes that must respond to a read request.
- W (Write Quorum): The minimum number of nodes that must acknowledge a write.
- Example: If
N = 3
,R = 2
, andW = 2
, the system can tolerate one node failure while still ensuring data consistency.
- Consistency Models:
- Strong Consistency: Guarantees that every read reflects the latest write.
- Example: In a banking system, account balance reads must always reflect the latest transaction.
- Eventual Consistency: Guarantees that, given enough time, all replicas will converge to the same value.
- Example: In a social media platform, a user’s post might not immediately appear to all followers but will eventually be consistent across all replicas.
- Strong Consistency: Guarantees that every read reflects the latest write.
- Inconsistency Resolution:
- Versioning: Each write operation creates a new version of the data, allowing the system to detect and resolve conflicts.
- Example: If two versions of a user profile exist, the system might merge them or select the latest based on a timestamp.
- Vector Clocks: Used to track the causal relationships between different versions of a key’s value, helping to resolve conflicts by understanding the order of operations.
- Versioning: Each write operation creates a new version of the data, allowing the system to detect and resolve conflicts.
- Failure Handling:
- Merkle Trees: Data structures that allow efficient comparison and synchronization of data across replicas by comparing hash trees of data segments.
- Example: Used in Cassandra to detect and repair inconsistencies between replicas.
- Data Center Outage: Data is replicated across multiple geographically distributed data centers to ensure availability even if one data center fails.
- Merkle Trees: Data structures that allow efficient comparison and synchronization of data across replicas by comparing hash trees of data segments.
System Architecture
- Coordinator Node:
- In systems like Dynamo, a coordinator node is responsible for managing read and write requests, ensuring that the appropriate replicas are accessed or updated.
- Decentralized Architecture:
- Nodes in the system are designed to have equal responsibilities, eliminating single points of failure and enabling the system to scale horizontally.
Write Path
- Commit Log:
- When a write request is received, it is first persisted in a commit log (write-ahead log) to ensure durability.
- Example: A new user registration is logged before being written to the in-memory database.
- Memory Cache:
- After being logged, the data is stored in an in-memory cache for fast access.
- Example: User session data is cached in memory for quick retrieval.
- SSTables:
- Once the in-memory cache reaches a certain threshold, the data is flushed to disk in the form of Sorted String Tables (SSTables), which are immutable and can be merged efficiently.
Read Path
- Memory Lookup:
- The system first checks if the data is in memory. If found, it is returned directly.
- Example: If a user profile is cached, it’s returned immediately.
- Bloom Filter:
- If the data is not in memory, a Bloom filter (a probabilistic data structure) is checked to quickly determine if the data might be in the SSTables on disk.
- Example: Before searching SSTables, the Bloom filter can suggest whether the data is likely present, reducing unnecessary disk I/O.
- SSTable Query:
- If the Bloom filter indicates a possible match, the relevant SSTables are queried to retrieve the data.
- Example: The system searches the disk-based SSTables if the data is not found in memory.
Real-World Examples
- Dynamo (Amazon):
- Dynamo uses consistent hashing for data distribution, a quorum mechanism for consistency, and vector clocks for conflict resolution. It is designed to prioritize availability and partition tolerance.
- Cassandra (Apache):
- Cassandra, inspired by Dynamo, is an AP system that supports eventual consistency, tunable consistency levels, and uses SSTables for efficient storage and retrieval of data.
- BigTable (Google):
- BigTable uses a distributed architecture, supports strong consistency, and employs SSTables and a hierarchical structure for managing large-scale data across multiple nodes.
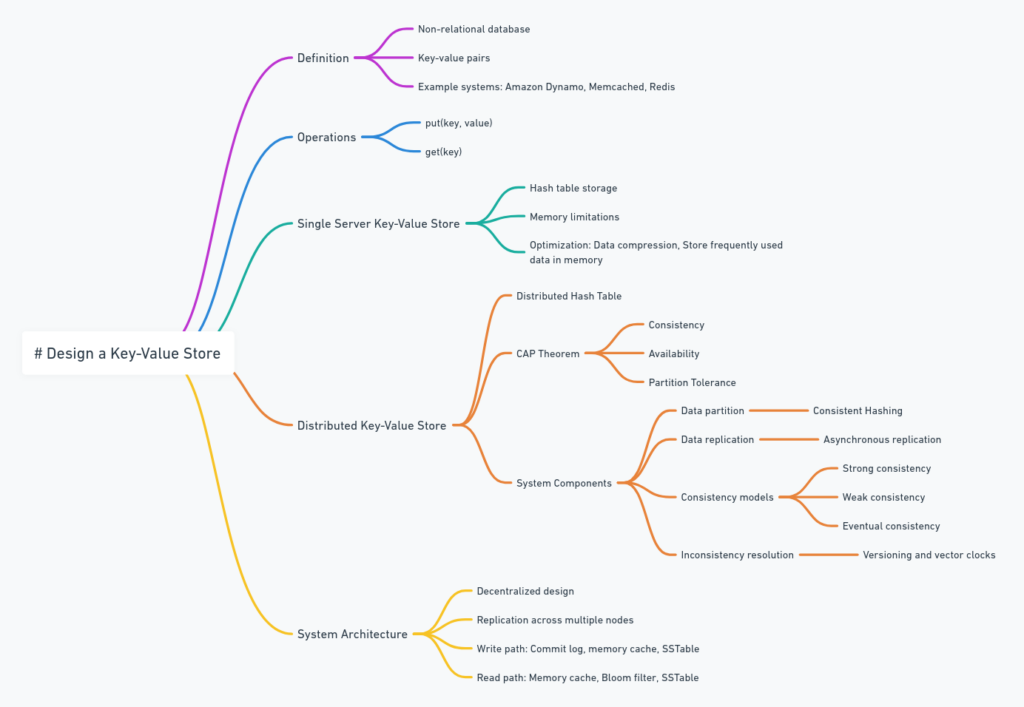
Conclusion
Chapter 6 provides a thorough understanding of the design principles behind key-value stores, balancing the need for high availability, scalability, and consistency. By exploring real-world systems like Dynamo, Cassandra, and BigTable, the chapter illustrates how these concepts are applied in practice, offering valuable insights for designing robust and scalable key-value stores in distributed environments.